Computational single-cell biology
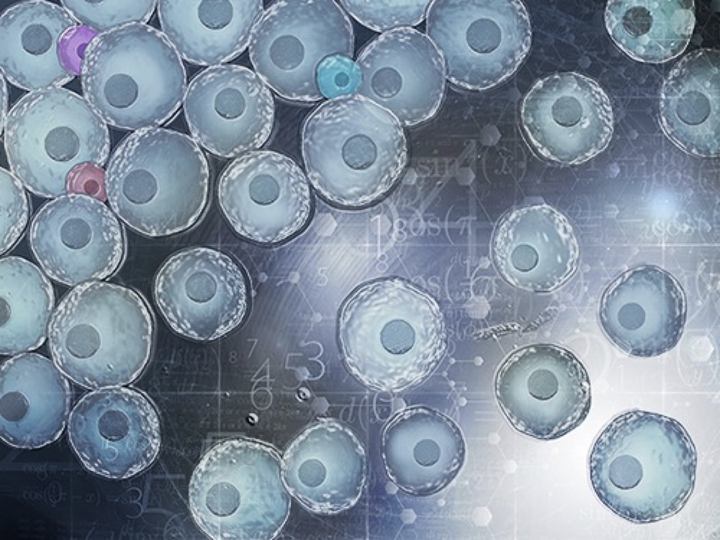
Single-cell RNA-seq
One research focus of MLO is on the development of application driven probabilistic machine-learning methods for analyzing high-throughput single-cell RNA-seq data. Single-cell RNA sequencing (scRNA-seq) has become a widely used routine assay and recent scRNA-seq protocols now allow to obtain an unbiased profile of transcriptional heterogeneity at a genome-wide scale, which can be scaled to large datasets with up to millions of cells. Analysis of such data is challenging: As the intrinsic cellular state of individual cells cannot be directly assayed, cell states need to be inferred from the transcriptional profiling data itself. To this end, single-cell profiling data pose new challenges and opportunities to derive powerful latent variable models (LVMs) that help to exploit large datasets.
Visualization of latent cell states
We have developed several tools tailored towards inferring and visualizing internal cell states. This includes inferring the internal cell-cycle state of individual cells via Gaussian Process Latent Variabe Models (GPLVM), as well as GPLVM-based identification of cell subpopulations. In addition, we have created tools based on diffusion maps that allow to recover the intrinsic order of cels, e.g. in terms of their differentiation state.
Identification of sources of cell-cell variation
Cell-cell variability in terms of gene expression results from a variety of potential sources, including technical noise, biological variation of interest and unwanted biologicl variation (e.g. mitochonrial genes, cell cycle state, etc.). To disentangle these sources of variation, we designed slalom, an interpretable latent variable model that addresses three key tasks in computational scRNA-seq
- Disentagling biological and technical sources of variation
- Visualizing the pathways driving cell-cell variation
- Refining pathway annotations in an experiment-specific manner