Multi-omics data integration
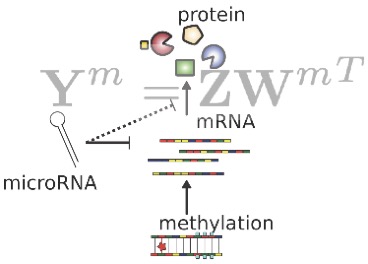
Multi‐omics studies promise the improved characterization of biological processes across molecular layers. However, methods for the integration of the resulting heterogeneous data sets are lacking. We develop tools and algorithms for the supervised as well as unsupervised integration of such data.
Unsupervised data integration
For example, we present Multi‐Omics Factor Analysis (MOFA), an unsupervised computational method for discovering the principal sources of variation in multi‐omics data sets. MOFA infers a set of (hidden) factors that capture biological and technical sources of variability. It disentangles axes of heterogeneity that are shared across multiple modalities and those specific to individual data modalities. The learnt factors enable a variety of downstream analyses, including identification of sample subgroups, data imputation and the detection of outlier samples. We applied MOFA to a cohort of 200 patient samples of chronic lymphocytic leukaemia, profiled for somatic mutations, RNA expression, DNA methylation and ex vivo drug responses. MOFA identified major dimensions of disease heterogeneity, including immunoglobulin heavy‐chain variable region status, trisomy of chromosome 12 and previously underappreciated drivers, such as response to oxidative stress. In close collaboration with the Oellerich group in Frankfurt, we use MOFA to elucidate the proteogenomic landscape in a range of cancers. In addition, we use MOFA to analyse single‐cell multi‐omics data, for example identifying coordinated transcriptional and epigenetic changes along cell differentiation and analysing the epigenetic landscape during mammalian germ layer specification.
Supervised analysis of multi-omics data
In addition to these unsupevised approaches, we also develop tools for integrative gene-set enrichment analyses in supervised multi-omics settings. In particular, our algorithm MONA and its accompanying webservice RAMONA use a Bayesian approach with a computationally efficient method to approximate the marginal posteriors of ontology terms, given lists of genes responding to experimental conditions. MONA is designed to easily handle any combination of molecular levels in a modular fashion.